12.05.16
Time to learn from the retail sector
Source: NHE May/Jun 16
Richard Edmondson, professor of gynaecological oncology at the University of Manchester, explains how a new scheme funded by Cancer Research UK will be able to extract and predict data to dramatically improve cancer treatment.
In contrast to Tesco, which learns something from each of its shoppers on every visit, the NHS learns nothing from the 80% of its cancer patients who start and finish their treatment without taking part in clinical trials.
Does this matter? Absolutely. The holy grail of cancer therapy remains the concept of personalised medicine in which the best treatment is given to each patient at the optimal time.
We can’t do that though without knowing three things about each patient before we start treatment: will the tumour respond; can the patient tolerate the treatment; and can the treatment centre deliver it effectively?
There has been tremendous effort to develop biomarkers, tests of the tumour to predict response, which will effectively answer the first question but the other two questions have largely been ignored.
However, the NHS is sitting on huge amounts of data for each and every one of its patients. These data are collected and used during diagnosis and treatment but are then stored away in one of many data silos where they seem to get lost.
Much of the information required to answer the questions above, though, is stored in these data collections including the past medical history of a patient, the results of previous blood tests and previous treatment outcomes. Many of these data items are already recognised as predictive but there are many more waiting to be discovered.
Machine learning algorithms
Accessing these data is not an easy matter and linking them back to each other can be difficult. However, within Manchester, as part of the devolution agenda, major initiatives are already underway to create federated databases that will link data held in primary and secondary care.
There is therefore a real potential to be able to extract and use these data to see if we can generate predictive algorithms that will improve therapy for patients and lead us toward the goal of personalised therapy.
Extracting and collating the data is only one of the challenges. How do we analyse all these disparate data when we get them? This is where we can really learn from the retail and commercial sectors where machine learning tools are a part of daily life.
Machine learning algorithms love data and can make sense of large databases even when significant parts are missing, something which really limits conventional models. As their name suggests they learn with time and data, which improves their accuracy and means they remain responsive to changes in treatment patterns.
Information on new drugs and data coming from the 100,000 genome project, for instance, could also be included for those patients where such data are available.
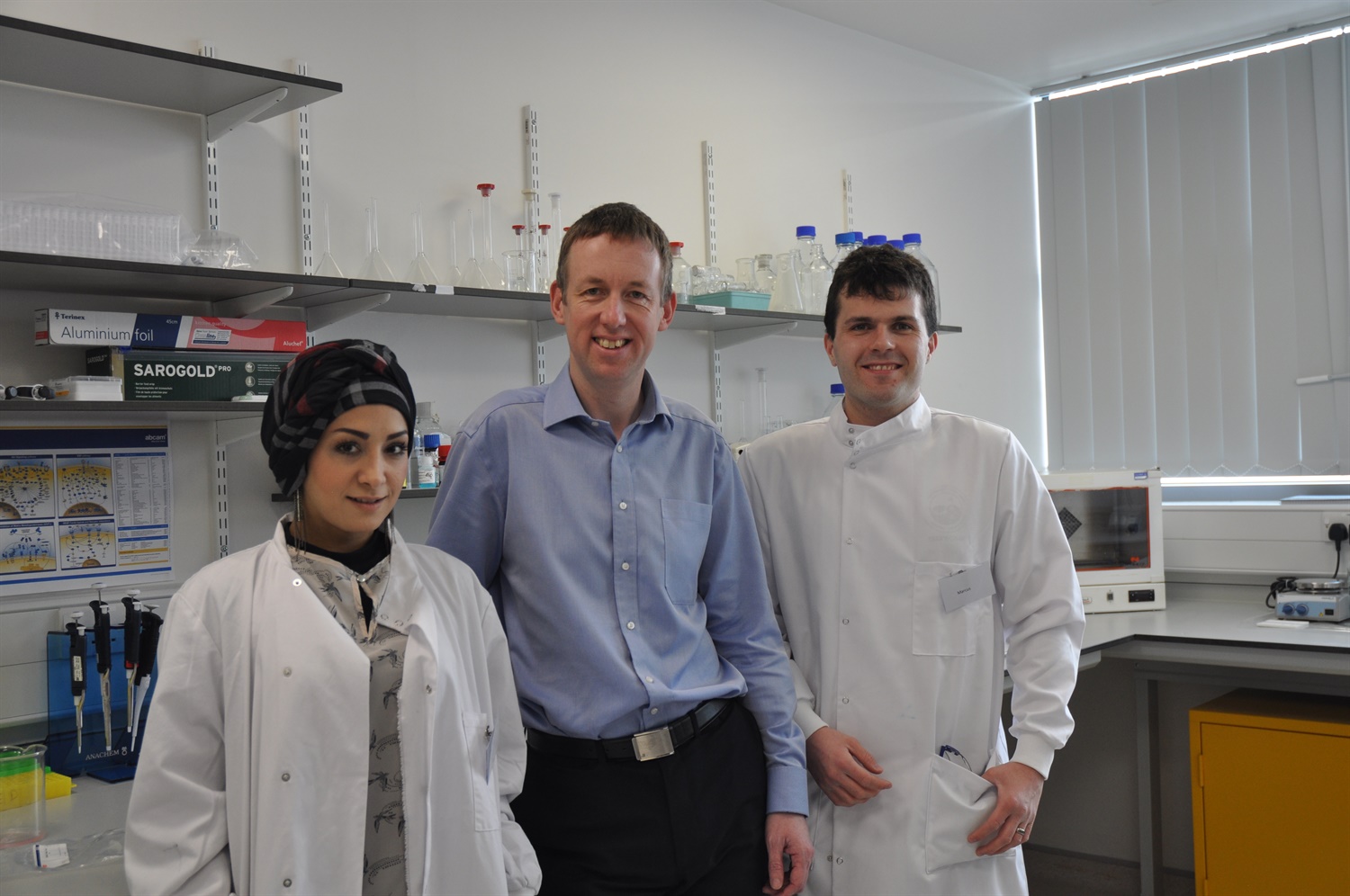
Predicting clinical outcomes
Our initial work in this area shows that machine learning tools outperform conventional statistical tools in their accuracy of prediction, and it is now time to apply this to larger datasets to attempt to answer real clinical questions.
Cancer Research UK is supporting this initiative through its Pioneer Grant Award Scheme. The funding it has provided will support our research team based at the University of Manchester to set up an automatic data extraction pipeline for patients in Greater Manchester with ovarian and bowel cancer and use our developed machine learning systems to analyse this.
Specifically, we want to develop a tool that will predict the outcome of surgery to enable us to avoid doing an operation with little chance of success and spare the patient the morbidity of such a procedure.
There are many other questions arising from this work that we also need to start answering such as: How accurate does a prediction need to be to make it clinically useful? Does a surgeon’s practice change when he starts using such a tool? Will patients find this sort of decision-making acceptable?
To answer these questions, Cancer Research UK is also funding a researcher to start working with patients and surgeons to assess the acceptability of such an algorithm. Ultimately, we hope to have a system that will deliver a predictive score to the clinician’s desktop by the time they are seeing the patient for the first time.
So maybe it won’t be too long before all those things we already know about patients are being collated, analysed and delivered to clinicians in a useful way to help them make the right decision.
Tell us what you think – have your say below or email [email protected]